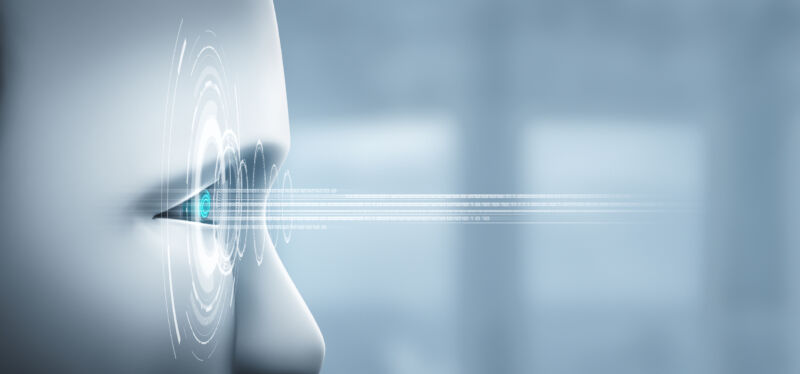
Getty Photos
Within the final couple of years, we have seen wonderful developments in AI methods in the case of recognizing and analyzing the contents of difficult photos. However a brand new paper highlights what number of state-of-the-art “imaginative and prescient studying Fashions” (VLMs) typically fail at easy, low-level visible evaluation duties which might be trivially simple for a human.
Within the provocatively titled pre-print paper “Imaginative and prescient language fashions are blind“ (which has a PDF model that features a darkish sun shades emoji within the title), researchers from Auburn College and the College of Alberta create eight easy visible acuity exams with objectively right solutions. These vary from figuring out how typically two coloured traces intersect to figuring out which letter in an extended phrase has been circled to counting what number of nested shapes exist in a picture (consultant examples and outcomes may be seen on the analysis workforce’s webpage).
-
When you can clear up these sorts of puzzles, you’ll have higher visible reasoning than state-of-the-art AIs.
-
The puzzles on the precise are like one thing out of Highlights journal.
-
A consultant pattern exhibits AI fashions failing at a process that almost all human kids would discover trivial.
Crucially, these exams are generated by customized code and do not depend on pre-existing photos or exams that might be discovered on the general public Web, thereby “minimiz[ing] the possibility that VLMs can clear up by memorization,” in response to the researchers. The exams additionally “require minimal to zero world information” past primary 2D shapes, making it tough for the reply to be inferred from “textual query and selections alone” (which has been recognized as a problem for another visible AI benchmarks).
Are you smarter than a fifth grader?
After working a number of exams throughout 4 completely different visible fashions—GPT-4o, Gemini-1.5 Professional, Sonnet-3, and Sonnet-3.5—the researchers discovered all 4 fell effectively in need of the one hundred pc accuracy you may count on for such easy visible evaluation duties (and which most sighted people would have little bother reaching). However the measurement of the AI underperformance diverse significantly relying on the precise process. When requested to rely the variety of rows and columns in a clean grid, as an illustration, the best-performing mannequin solely gave an correct reply lower than 60 % of the time. However, Gemini-1.5 Professional hit practically 93 % accuracy in figuring out circled letters, approaching human-level efficiency.
-
For some motive, the fashions are likely to incorrectly guess the “o” is circled much more typically than all the opposite letters on this check.
-
The fashions carried out completely in counting 5 interlocking circles, a sample they could be conversant in from widespread photos of the Olympic rings.
-
Do you could have a better time counting columns than rows in a grid? In that case, you most likely aren’t an AI.
Even small modifications to the duties might additionally result in large modifications in outcomes. Whereas all 4 examined fashions had been in a position to appropriately establish 5 overlapping hole circles, the accuracy throughout all fashions dropped to effectively under 50 % when six to 9 circles had been concerned. The researchers hypothesize that this “means that VLMs are biased in direction of the well-known Olympic brand, which has 5 circles.” In different instances, fashions often hallucinated nonsensical solutions, equivalent to guessing “9,” “n”, or “©” because the circled letter within the phrase “Subdermatoglyphic.”
Total, the outcomes spotlight how AI fashions that may carry out effectively at high-level visible reasoning have some vital “blind spots” (sorry) in the case of low-level summary photos. It is all considerably harking back to comparable functionality gaps that we regularly see in state-of-the-art massive language fashions, which may create extraordinarily cogent summaries of prolonged texts whereas on the similar time failing extraordinarily primary math and spelling questions.
These gaps in VLM capabilities might come right down to the lack of those methods to generalize past the sorts of content material they’re explicitly educated on. But when the researchers tried fine-tuning a mannequin utilizing particular photos drawn from one in all their duties (the “are two circles touching?” check), that mannequin confirmed solely modest enchancment, from 17 % accuracy as much as round 37 %. “The loss values for all these experiments had been very near zero, indicating that the mannequin overfits the coaching set however fails to generalize,” the researchers write.
The researchers suggest that the VLM functionality hole could also be associated to the so-called “late fusion” of imaginative and prescient encoders onto pre-trained massive language fashions. An “early fusion” coaching strategy that integrates visible encoding alongside language coaching might result in higher outcomes on these low-level duties, the researchers counsel (with out offering any type of evaluation of this query).